AI at Smart Refinery
Tomi Lahti, Product Manager / 29 Apr 2021
Today we see how Industry 4.0 is delivering value for process industries with the Smart Refinery concept. Data is the fuel, data management, governance and related systems are the fuel system. But the true engine is AI. It can optimize production processes, but above all AI is to support human decision making and situational awareness.
Situational awareness is familiar from aviation. The plane could fly automatically, but the pilot is still retained as the main decision-maker. In production, the same situational awareness is the key to the safe process operations in situations where something unexpected happens. Safety is the reason why various complex production processes cannot be fully autonomous.
Therefore, human presence will have an essential role for safety in the age of AI also. To make disruption and deviation management as smooth as possible, AI and human operators need to work together; The role of AI is to support daily human decision making and at the same time keep the person informed of the situation (human-in-the-loop like in aviation). Human-machine inter-operation is in the center of Industry 4.0. At Smart Refineries this is referred to as the concept of Operator 4.0. Because the role of technology is to support people and help humans to reach their full potential.
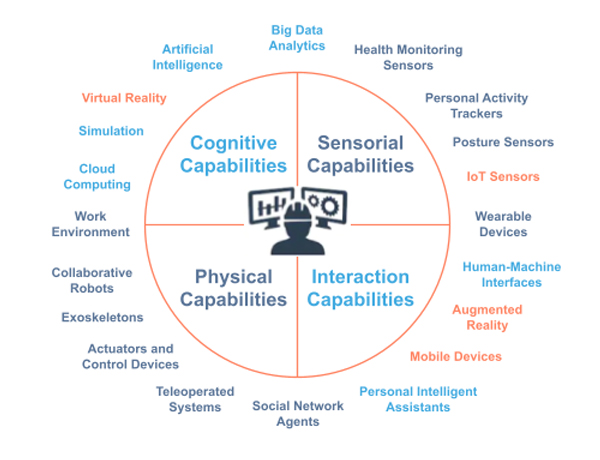
While AI supports humans, data based machine learning models can also be utilized in the broader optimization of refinery operations, along with other, more traditional forecasting models.
Supply chain and refinery material flows need to obey customer needs, market demand as well as utility and feedstock pricing. Particularly, internal material flows like blending and loading of vessels benefit from predictions available from production digital twins, and are essential for optimal production planning and scheduling.
Digital Twins of production and supply chain are essential elements for plant-wide optimization and overall supply chain optimization. Another important solution area for data based models is predictive maintenance. By reliably estimating the correct time frame for maintenance beforehand (or running assets so that maintenance can be delayed to a later date, for example due to customer demand) improves efficiency and reliability.
All of these models require reliable streaming data to be able to predict the future and to serve a common goal, optimal production which minimizes costs and GHG emissions and maximizes flows of most valuable products at each moment of time. Answer is to build a proper ML Pipeline to serve all these use cases. Next time more about that.